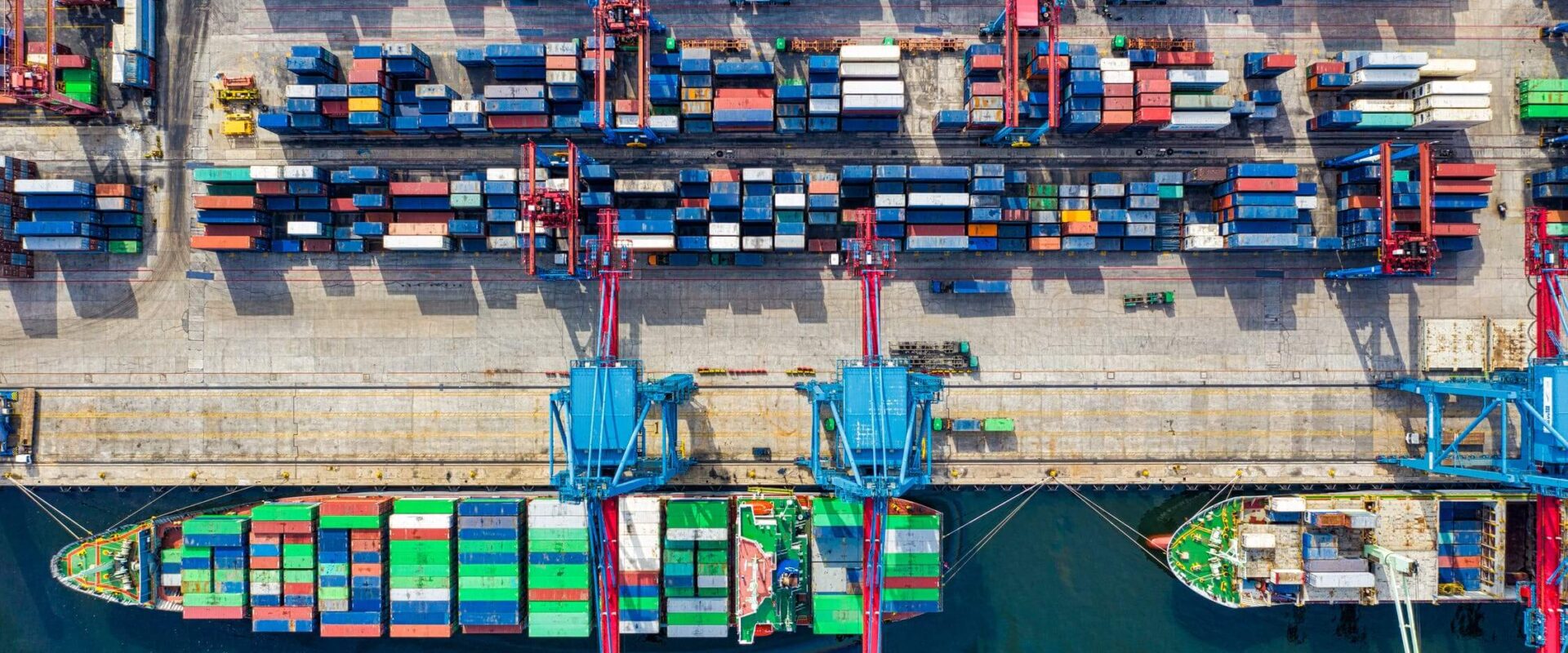
AI FOR THE SUPPLY CHAIN
Prediction
One of the most challenging aspects of managing a supply chain is predicting future demand. Without AI, this is done by human subjective judgments, with hit and miss results. Using machine learning algorithms to analyze large, diverse datasets drastically improves the accuracy of demand forecasting. For example, a regression algorithm can predict future demand based on all known variables, such as the time of year, previous demand or competitor sales. It can also reduce under-supply when demand is low and reduce waste when it’s high.
Planning optimization
AI can determine the optimum schedule for deliveries. Final delivery of goods might, for example, require a driver to leave a depot, circle around several locations and return. Traditional algorithms for this problem are slow and do not allow for other possibilities, such as multiple drivers or prioritized items. AI offers several approaches to optimize route planning from deep reinforcement learning solutions to swarm intelligence and evolutionary algorithms.
Supplier risk management
AI can analyze datasets, such as assessments, audits and credit scoring, which provide an important basis for further decisions regarding a supplier. Supplier selection can be more predictive than ever before. Machine-to-machine automation provides multiple “best supplier” scenarios based on your specific parameters.
End-to-end visibility
Combining machine learning with advanced analytics, IoT sensors and real-time monitoring is providing end-to-end visibility for the first time across many supply chains. What’s needed in many supply chains today is an entirely new operating platform or architecture predicated on real-time data, enriched with patterns and insights not visible in the past with previous analytics tools. Machine learning is an essential element in future supply chain platforms that will revolutionize every aspect of supply chain management.
Robotics
Robots, such as Amazon’s Kiva robots, can pick up goods and distribute them to different stations within a warehouse in mere minutes, and they only need five minutes every hour to charge. The robots lift and stack plastic bins full of products, then they take the products to pickers, who select the right product for shipment.
Automated supply chain
The future is an automated supply chain, which:
- Predicts demand to precisely match supply
- Optimizes planning to identify the optimal routes
- Reduces supplier risks by analyzing unstructured data
- Combines with IoT for automated monitoring and quality assurance
- Delivers real-time, end-to-end visibility
- Replaces human beings with robots, who work without breaks in warehouse fulfillment
All this is happening before self-driving cars completely revolutionize logistics. By 2040, self-driving cars will account for 95 percent of all vehicles sold. As the often-quoted British supply chain guru Dave Waters says, “Artificial Intelligence will permeate supply chains in ways we haven’t even thought of.”
CASE STUDY: AI FOR SUPPLY CHAIN SCHNEIDER ELECTRIC’S GLOBAL SUPPLY CHAIN
Challenge
Schneider wanted to reduce the costs involved in the company’s existing supply chain flows for 240 manufacturing facilities around the world and 110 distribution centers. It also wanted to analyze potential opportunities to assimilate new business units that Schneider had just acquired.
Approach
LLamasoft built a supply chain predictive model that could automatically create the best routing options for Schneider’s enormous raw materials supply chain, which includes circuit breakers that are small enough to fit on a store shelf to transformers that are the size of a large room. Data engineers at Schneider first built a data extraction tool that could collect the enterprise data from all the ERP systems, verify and “clean” the data to be input onto the LLamasoft platform.
Outcomes
The customized model needed about two to four hours to analyze 200,000 transportation policy data points, 130,000 flow and routing constraints, and more than 150 initial scenarios (data provided by Schneider). It identified $9.32 million in annual savings for Schneider, which could potentially be obtained by altering product flow in the supply chain. This might include, for example, rerouting certain shipments directly from the manufacturing plants, rather than through one of the distribution centers, to save on material handling and inventory storage costs.