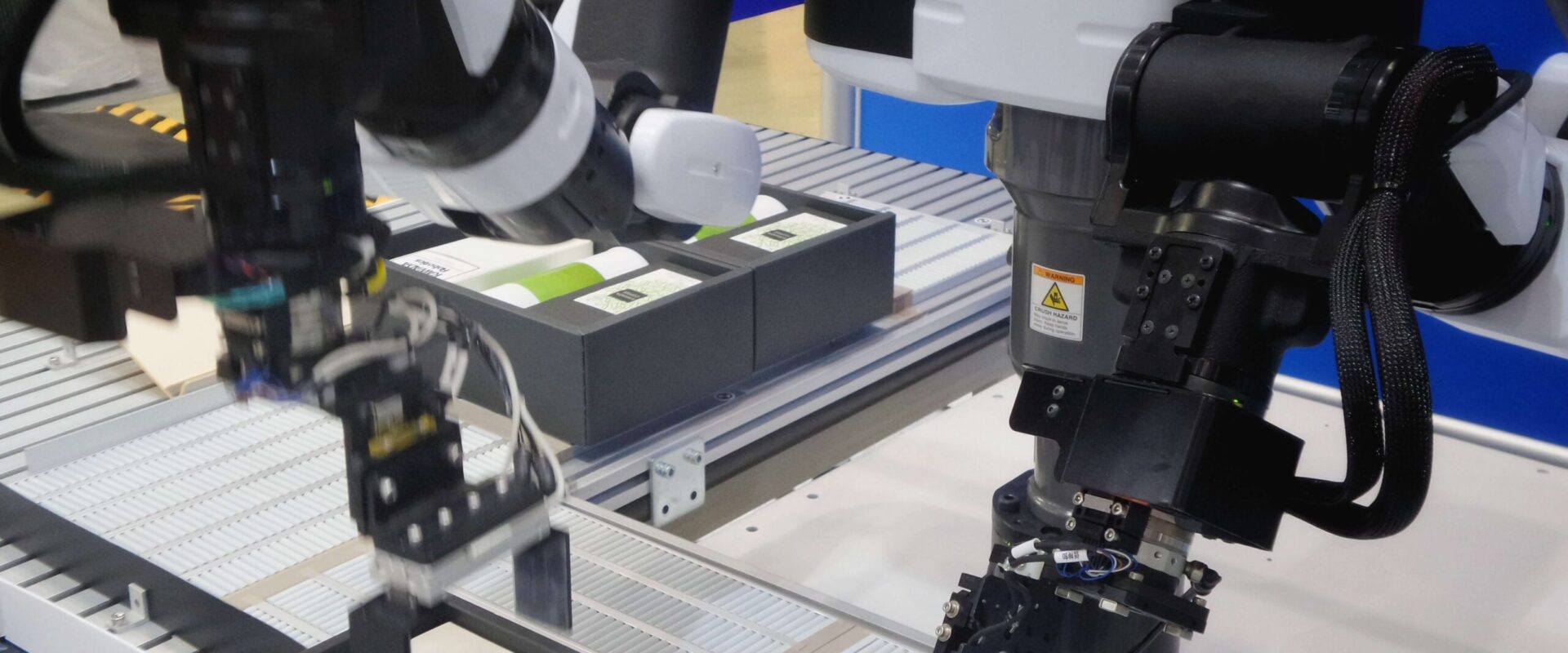
AI FOR OPERATIONS
Preserve energy
Preserving energy leads to a reduction in fixed line costs, but it’s also a CSR initiative. In utilizing AI to reduce energy consumption, your business also supports sustainability. Predictive asset utilization uses AI to preserve power only when you need it.
Predict maintenance
The cost of machine downtime is estimated at a staggering $647 billion lost globally each year, according to the International Society of Automation. Using AI to predict maintenance can prevent issues before they exacerbate and, in the process, lower costs of scheduled maintenance.
Predictive maintenance uses deep learning to analyze historic data to develop models to predict when a machine might break down or otherwise stop performing. This can feed into a control system that works in real time for interventions aimed at reducing downtime and increasing operational efficiency.
Quality and safety management
AI for production lines includes machine vision inspection systems to maintain quality and safety. Landing.ai, founded by Andrew Ng, uses machine vision to find microscopic defects in products at resolutions beyond human vision. The machine is able to recognize unknown defects and send alerts for “automated issue identification.”
Automating manufacturing operations
One of the main drivers of manufacturing productivity has been the introduction of robot workers. Invigorating these robots with AI offers the opportunity to improve workflow efficiency, flag potential risks and adapt to changing circumstances. These advantages of AI-driven automation offer improvements in the use of time and resources. Over time, AI-fuelled robots can learn to work more effectively with their human counterparts.
Planning and scheduling
Using AI for planning and scheduling enables you to predict which processes, people and tools will deliver optimum productivity. AI can allocate appropriate personnel, automate the scheduling of tasks, have real-time management insights and dashboards, and accurately predict project timelines based on historic data and real-time performance.
Automatically scheduling jobs with AI uses machine learning combined with rules and parameters set by the production manager. AI algorithms make the various routine, real-time manufacturing scheduling decisions that are needed in a modern manufacturing plant. Human beings remain in the loop, providing useful oversight of the machine schedulers.
CASE STUDY: AI FOR OPERATIONS GOOGLE DEEPMIND ASSET UTILIZATION2
DeepMind implemented deep learning to reduce energy costs and waste in Google data centers. The company found that “reducing energy usage has been a major focus for us over the past 10 years.
. . . One of the primary sources of energy use in the data center environment is cooling. Just as your laptop generates a lot of heat, our data centers—which contain servers powering Google Search, Gmail, YouTube, etc.—also generate a lot of heat that must be re-moved to keep the servers running,” says DeepMind. Implementing machine learning enabled DeepMind “to reduce the amount of energy used for cooling by up to 40 percent.” This equated to “an over-all 15 percent reduction in overall PUE overhead after accounting for electrical losses and other non-cooling inefficiencies,” according to DeepMind. The implications for deep learning go further than Google’s data centers, “given its potential to greatly improve energy efficiency and reduce emissions overall,” which helps Google address climate change and sustainability issues.
- “DeepMind AI Reduces Google Data Centre Cooling Bill by 40%,” Deepmind.com, July 20, 2016, https://deepmind.com/blog/deepmind-ai-reduces-google-data-centre-cooling-bill-40/.
- “DeepMind AI Reduces Google Data Centre Cooling Bill by 40%,” Deepmind.com, July 20, 2016, https://deepmind.com/blog/deepmind-ai-reduces-google-data-centre-cooling-bill-40/.